
Introduction
Organizations need data processing to reap the advantages of quality data. The processed data can provide them with a competitive edge and deliver critical business insights to drive correct decisions. Hence, fast-growing organizations ensure the quality of data processing of their data consciously and treat it as a critical part of their operations.
What is data processing?
Data processing occurs when unorganized, raw data is collected and translated into usable information. Data engineers and Data Scientists usually perform it. It is essential for data processing to be done correctly as low-quality data can negatively affect the end product or data output.
The process starts with working on data in its raw form and converting it into a more readable format (text, infographics, charts, documents, etc.). Giving data the form and necessary context to be interpreted by computers and utilized by employees throughout the organization.
What are the stages in Data processing?
Data processing comprises six primary steps:
Data Collection
Data Preparation
Data Input
Core Processing
Data Output/Interpretation
Here's the detailed explanation
Data Collection
The first step to processing data starts by collecting it from various available sources. Data is pulled from sources called data lakes and data warehouses. Maintaining data quality and ensuring that available data sources are trustworthy and well-built is vital as data quality determines the final usability and accuracy of the driven insights.
Data Preparation
The collected data enter the next stage of data preparation, referred to as ‘pre-processing.’ In this stage, raw data is cleaned up and organized for the further stages of data processing. Raw data is attentively checked for any errors and the presence of unwanted data. This step is performed to eliminate any bad data (that is redundant, incomplete, or incorrect data) and create high-quality data sets for processing.
Data Input
The cleaned-up data is then fed into its destination, perhaps a CRM like Salesforce or a data warehouse like Redshift. This process of feeding data is known as data entry. Translation into a language that the destination tool can understand could be a preliminary step. Data input is the first stage when raw data starts to take the form of organized usable information.
Processing
The data fed to the computer in the previous stage is now actually processed for interpretation. Machine learning algorithms process this data. However, the method may vary slightly depending on the data source, like social networks, connected devices, etc. This process may also vary depending on the data’s intended use, such as examining advertising patterns, medical diagnoses, determining customer needs, etc.
Data Output/Interpretation
In this stage, the processed data is finally usable to non-data scientists. It is translated and has improved readability and often is in the form of graphs, videos, images, plain text, etc. This data is now available for the organization to begin self-serve for their own data analytics project.
Data Storage
Storing the processed data is the final stage of data processing. When all the data is processed, it is reserved for future use. There could be a situation when the data is put to use immediately. However, much of it will serve the purpose later. Also, appropriately storing data becomes necessary to comply with data legislation processes like GDPR. When data is stored in an organized way, it can be quickly and easily accessed by members of the organization when needed.
The future of data processing
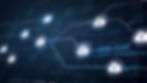
The future of data processing lies in cloud technology. Cloud helps build on the convenience of current electronic data processing methods while accelerating its speed and effectiveness. High-quality data with flexible access means more data for organizations to utilize and extract more valuable insights.
Organizations have tremendous benefits as they migrate big data to the cloud. Big data cloud technology allows companies to combine data from all platforms into a quickly-adaptable system. Software often updates (as it is more often when it comes to big data tools), and cloud technology seamlessly integrates the old with the new.
The benefits of cloud data processing are not just limited to large organizations. Small organizations can also reap most of the cloud’s benefits. Cloud platforms provide the flexibility to grow and expand capabilities as the organization grows while being affordable at the same time. It gives companies to scale without substantial price tags.
From data processing to analytics
Big data has changed the way the world used to do business. Today, the competition depends on having a clear and compelling data processing strategy. While the steps to process the data remain the same, the cloud has driven considerable technological advances that offer the most advanced, cost-effective, and fastest data processing methods to date.
Sakhsham, your data processing partner.
Sakhsham serves businesses in 7 countries as an expert data processing partner in their business expansion journey. Check out our Data Processing Services here.
Ready to discuss your next data processing project?